Coconut Palm Tree Detection in Samar Island using SkySat Imagery Through Advanced Deep Learning Identification
By Jeremy Ricofuerto, Assistant Remote Sensing Specialist
Geospectrum Remote Sensing | Jan 14, 2025
READ TIME: 9 MINUTES
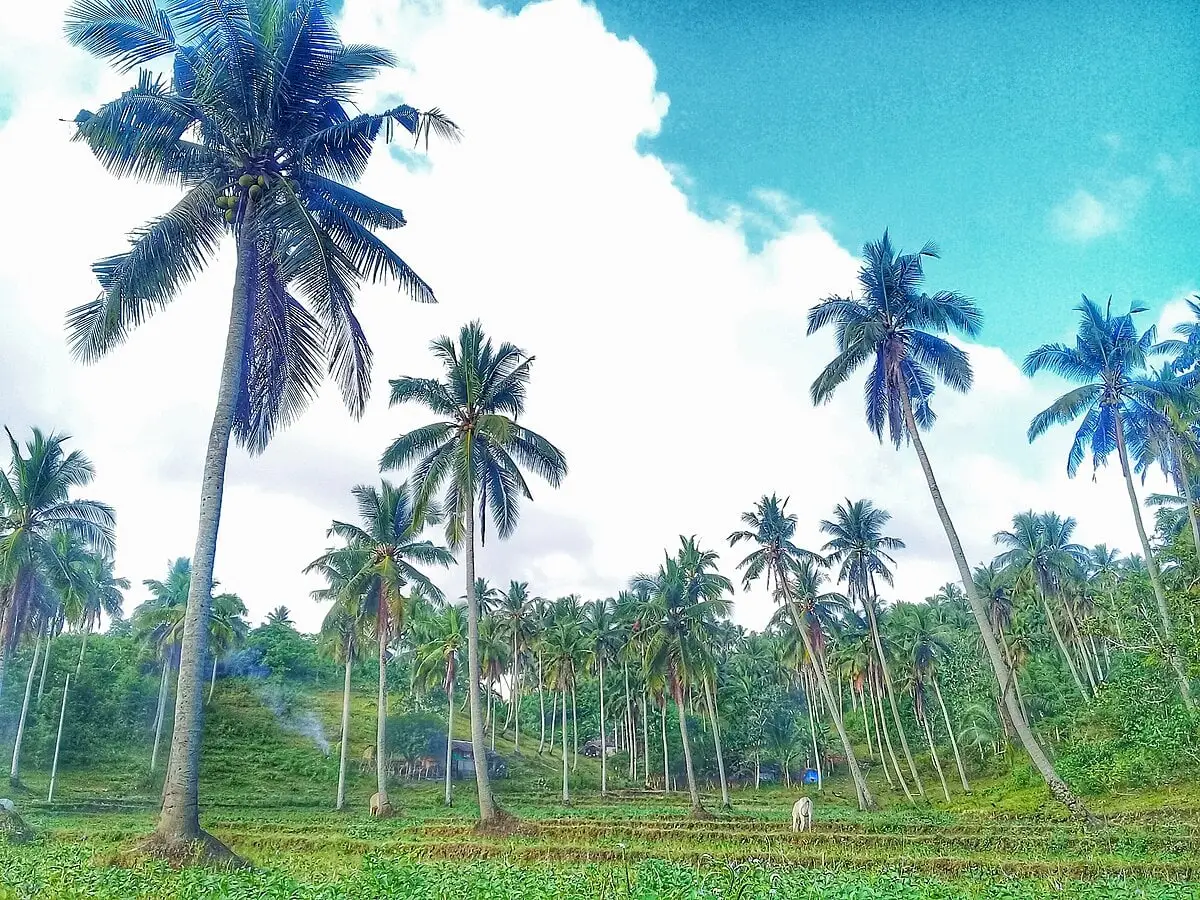
Figure 1: Coconut Plantation in Sipocot, Camarines Sur Image Source: (Creative Commons, 2018) Ships&Ports (2017).
For generations, the coconut, which is often referred to as the "tree of life,"
has been an essential component of Philippine agriculture. It supports livelihoods, offers products
with versatility, and contributes to the nation’s economy. But despite its importance to culture and
the economy, the coconut sector faces a variety of difficulties that have an influence on output and
the lives of coconut farmers.
The coconut industry in the Philippines has been struggling over time. The Philippines is currently
ranked second in the world behind Indonesia, despite having once led the world in coconut
production. India is also catching up quickly. The Philippines’ standing in the global market is
currently in jeopardy unless measures are taken to reverse the coconut industry’s downward
trajectory.
The coconut industry in Calbayog City, Samar has faced challenges due to the aging of coconut trees, leading to decreased productivity. This trend mirrors the national situation, where the Philippine coconut sector has experienced stagnation attributed to the advanced age of fruit-bearing trees, adverse weather conditions, and the impacts of climate change.
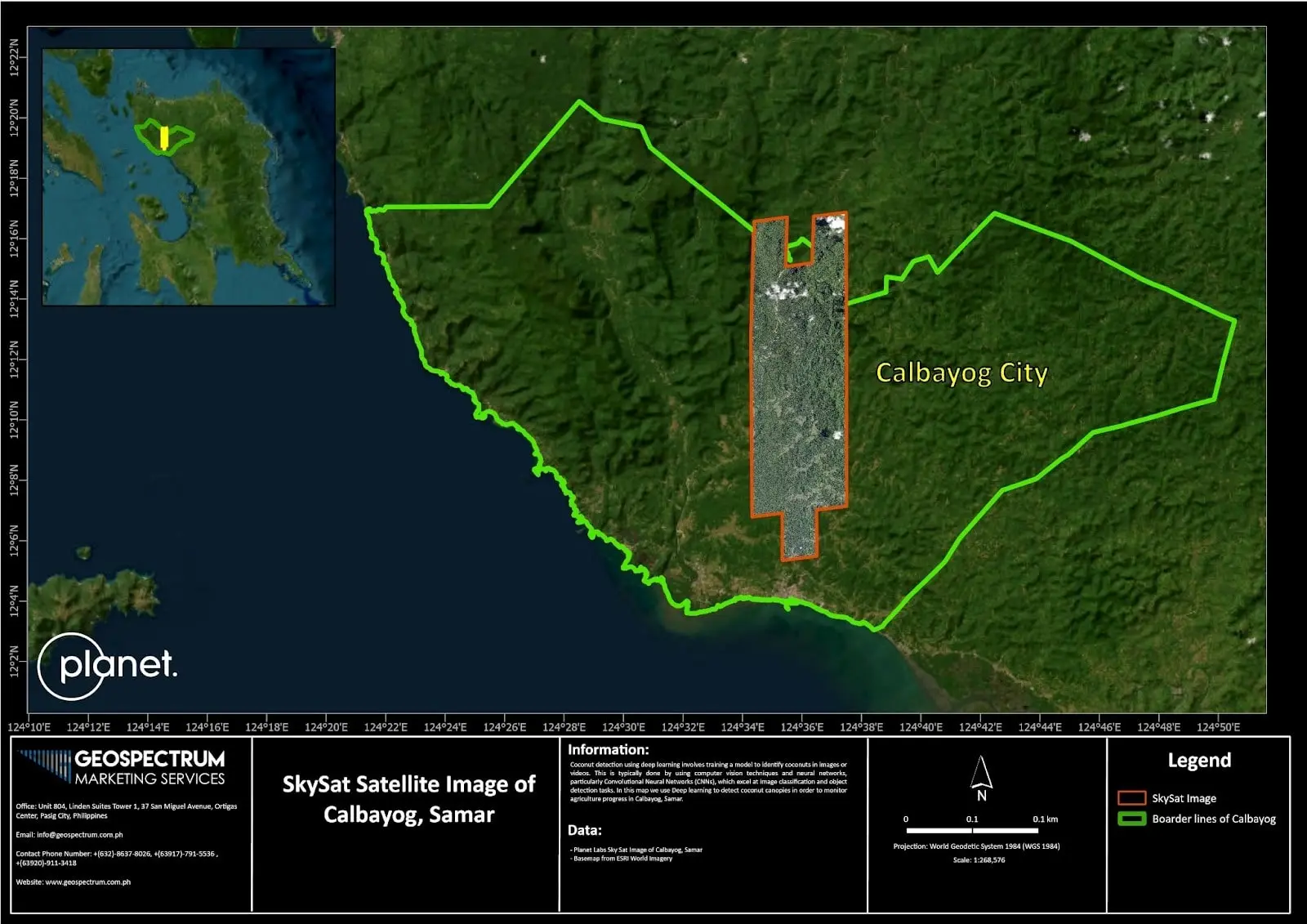
Figure 2: Preview of SkySat Satellite Image of Calbayog, Samar
Calbayog, Samar was chosen as the Area of Interest (AOI) for this article because Coconut is a major
agricultural commodity in Samar, Philippines, along with rice. Samar also is one of the main
producers of Coconut; where they have the Samar Coco Products Manufacturing Corporation. This
company is based in Calbayog and produces refined and crude coconut oil, as well as copra cake and
Coconut Fatty Acid Distillate (CFAD). The company has two factories in the Central and Southern
Philippines.
Samar also has programs that aim to improve the coconut industry and assist farming families by
providing capacity-building activities for farmers and food processors, such as the Coconut Farmers
and Industry Development Plan (CFIDP) and Coconut Hybridization Program.
Modern Tools for Identifying Diseased Coconut Trees
Geospectrum Marketing Services (GMS) developed a Deep Learning process in aid of the Coconut industry in Calbayog, Samar. Helping coconut tree growers confront the challenges and opportunities in the future. Below is the methodology GMS created:

Figure 3: A flowchart of training a Deep Learning Model for Coconut Detection in ArcGIS Pro
Tools for automatically identifying and recognizing plant diseases have shown themselves to be a useful source of information that supports agricultural decision-making. The increasing use of artificial intelligence techniques such as convolutional neural networks (CNN) and deep learning are two examples of artificial intelligence techniques that are becoming more and more popular in this industry since they offer the best solutions for identifying plant diseases.
Why use Deep Learning for Coconut Tree Detection
Currently, advances in geospatial analysis in agriculture in general has made the
continuous monitoring of crop growth possible through automation. This has increased crop quality
and offers the possibility of reduced labor costs by using vision-based techniques to detect and
pick up objects and using visual information for harvesting. (Bac et al., 2014)
Precision and modern agriculture are always changing, and technology is now essential to increasing
agricultural yields and shielding plants from pests and dangerous insects. A recent development in
contemporary agriculture is the application of neural networks, which allow robots to learn from and
identify patterns in data.
Real-time object detection technology is built on top of image processing software. The color, size,
texture, form, lighting, and reflectance qualities of the coconut present obstacles in the creation
of a quick and accurate machine vision image analysis method for its detection. Occlusions and
shadows in the tree crown are a critical concern as well, as they can greatly impede target
recognition.
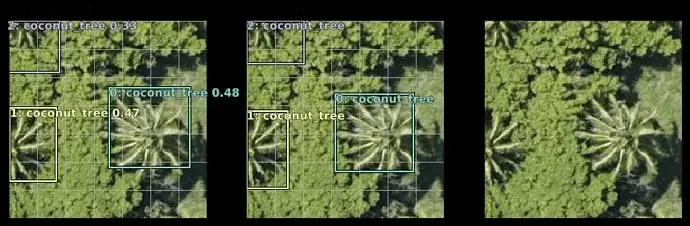
Figure 4: Detecting Coconut Trees using Satellite Image. Image Source: Forums, 2018.
Coconuts are commonly harvested by judging their maturity based on color, shape, timeframe, shaking
sound, and other growth characteristics of changes as they grow.
The detection of the maturation stages of coconuts for harvesting, without human intervention,
involves challenges because of the complexity of the environment and the similarity between fruits
and their backgrounds. (Parvathi, 2021)
Convolutional Neural Network (CNN) is a popular state of the art neural network architecture
efficacious in computer vision applications (Kieffer et al., 2018). Typically, CNN is hand-designed
for optimum accuracy for a specific application. In this application CNN is modified to classify
images from a satellite to identify coconut canopies. In this article, an improved Faster
Region-Based Convolutional Neural Network (Faster R-CNN) model was utilized for the detection of two
important maturity stages for coconuts in complex backgrounds.
Image Preprocessing
Detecting and counting trees in high-resolution images is essential for biomass estimation, environment, monitoring, and yield prediction. The coconut tree is one of the most important tree species, valued for its economic and material properties. Faster R-CNN is a basic object detection method, and has been applied in several tree crown detection studies
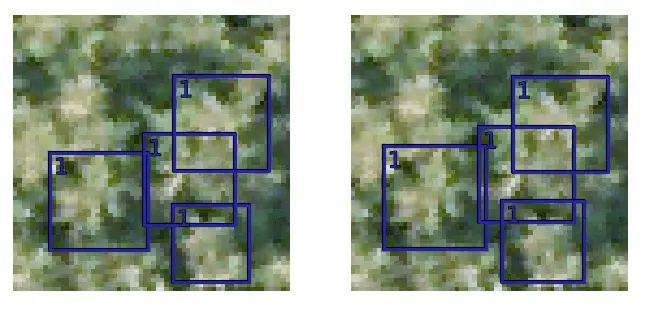
Figure 5: Ground Truth and Predictions output by Trained Image Chips created for the Deep Learning Model in ArcGIS Pro
We created shapefiles and modified training samples for a deep learning model using ArcGIS Pro.
What we did and found out
The Deep Learning Model that was trained shows a promising output, GMS re-trained the deep learning model using the existing exported image chips and added the max epochs giving the software 7 days in processing the model, the parameters used were adjusted.
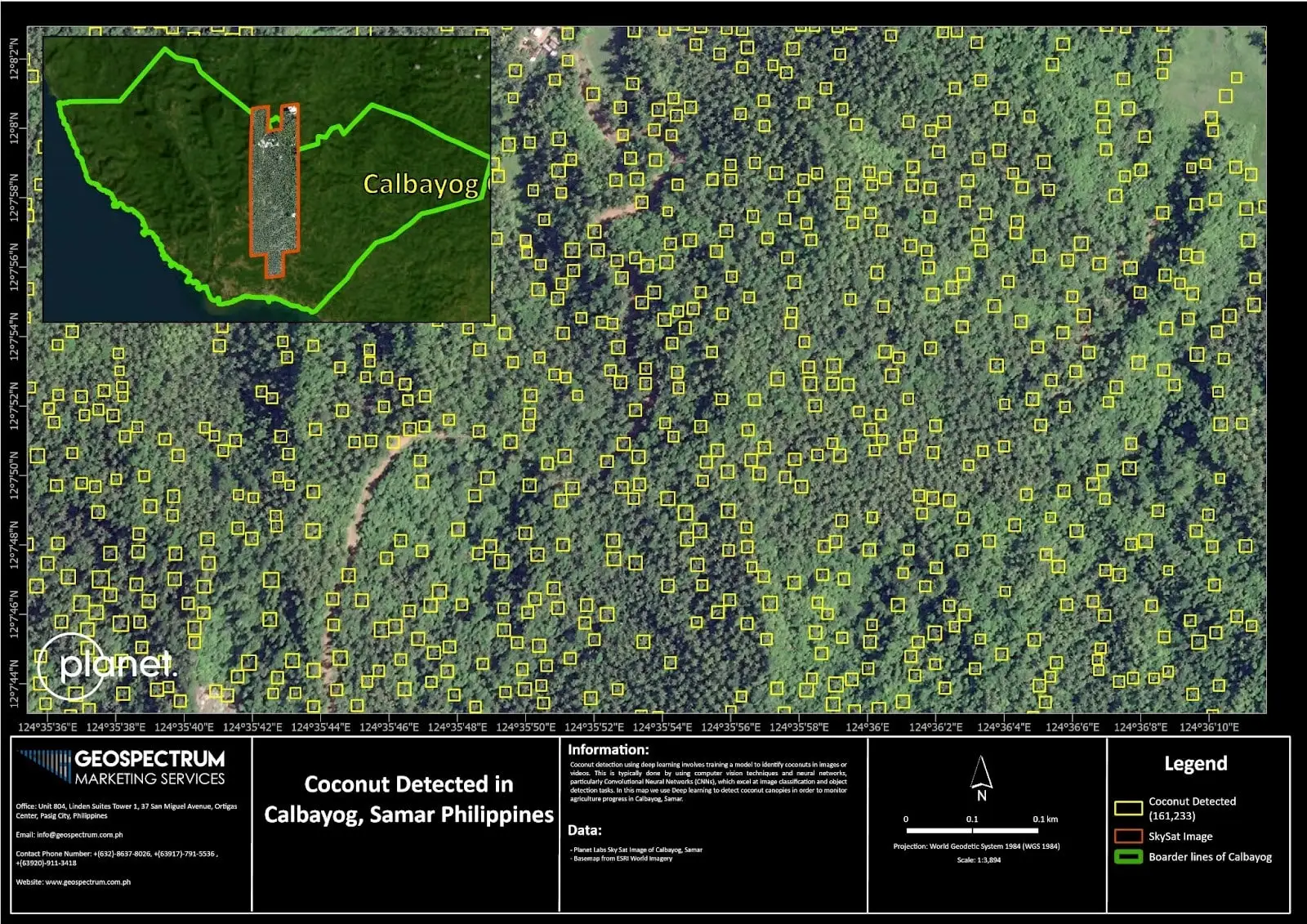
Figure 6: Final output of coconut detection in Calbayog, Samar
Factors like batch size, number of shapefiles and number of epochs were considered in improving the model. The first model trained has almost 11,195 training samples resulting in having a total of 161,233 detection of coconuts. The only disadvantage in training these samples into deep learning models is that it took 7 days to process. However, considering the time, cost, and manpower needed to conduct rapid field inspections of these essential trees makes this process a considerable alternative to the traditional counting process. Refer to Figure 5 above for the coconut trees detected in Calbayog, Samar using Deep Learning.
How can this developed application help you?
Using these remote sensing analytics that we have developed can be incredibly
beneficial in various domains such as:
- Management of Coconut Farms
- Coconut Tree Health Assessment: Use daily satellite images to track the health of coconut trees by identifying pests, disease, or nutrient deficits.
- Coconut Tree Growth Monitoring: Monitor the stages of growth to ascertain the best times for harvesting or evaluate the effects of fertilization and other interventions.
- Estimating Yield
- Improve supply chain planning and market forecasting by using machine learning models and remote sensing data to more precisely estimate coconut yields.
- Optimization of Resources
- Water Management: To maximize inputs to irrigation, pinpoint regions that are under water scarcity.
- Fertilizer Application: Identifies nutrient deficits and suggests accurate fertilizing schedule
- Planning and Land Use
- Crop Mapping: To differentiate coconut plantation sites from other land uses.
- Expansion Site Suitability Planning: Use soil and environmental data to determine the best locations for growing coconuts.
- Climate Impact Assessment
- Temperature, precipitation, and extreme weather occurrences are among factors considered while monitoring coconut productivity.
Are you curious about how our analytics can support your goals? Contact us through email at [email protected] or contact us at +(632) 8637-8026 or +(63917)-791-5536 to learn more about ship detection and PlanetScope imagery. You can also check out our website at www.geospectrum.com.ph to learn more about the products and services we are offering.
References:
Cabili, T. M. (2021). Environmental Conservation Mechanisms Of Small-Island In Capul, Northern Samar. In International Journal of Aquatic Science (Vol. 12). Cabili,2010. (n.d.). Ezequiel, C. A. F., Cua, M., Libatique, N., Tangonan, G., Honrado, J. L., Favila, C., Novilla, J., Ilaga, E. J., Alampay, R., Canos, V., Labuguen, R., Devaney, C., Loreto, A., Bacusmo, J., Lasquites, J. J., Bencure, J. C., Palma, B. A., & Raga-As, M. (n.d.). Aerial Imaging Consortium: Augmenting Ground Observation With UAS Remote Sensing for Environmental Resource Management. http://philgrss.org/journal/ Kareem, H. H., Omran, Z. A., & Attaee, M. H. (2023). Detection the Impact of Chlorophyll Index and Global Environmental Monitoring Index on Water Separation in Swansea in Wales, United Kingdom Through Analysing the Spectral Wavelengths of Landsat 8-OLI. Ecological Engineering and Environmental Technology, 24(9), 259–270. https://doi.org/10.12912/27197050/174007 Leprieur, C., Verstraete, M. M., & Pinty, B. (1994). Evaluation of the performance of various vegetation indices to retrieve vegetation cover from AVHRR data. Remote Sensing Reviews, 10(4), 265–284. https://doi.org/10.1080/02757259409532250 Parvathi, S., & Tamil Selvi, S. (2021). Detection of maturity stages of coconuts in complex background using Faster R-CNN model. Biosystems Engineering, 202, 119–132. https://doi.org/10.1016/j.biosystemseng.2020.12.002 Pogosa, J., Asio, V., Bande, M., Bianchi, S., & Grenz, J. (2018). Productivity and Sustainability of Coconut Production and Husk Utilization in the Philippines: Coconut Husk Availability and Utilization. In IJERD-International Journal of Environmental and Rural Development. Zheng, J., Wu, W., Yu, L., & Fu, H. (2021). COCONUT TREES DETECTION ON THE TENARUNGA USING HIGH-RESOLUTION SATELLITE IMAGES AND DEEP LEARNING. International Geoscience and Remote Sensing Symposium (IGARSS), 6512–6515. https://doi.org/10.1109/IGARSS47720.2021.9555008 SPDA Southern Philippines Development Authority Basilan coconut farmers decry ‘cocolisap’ infestation https://spda.gov.ph/basilan-coconut-farmers-decry-cocolisap-infestation/